How Are Enzyme Activity Assays Used for Drug Design
Key Points
-
The application of detailed mechanistic enzyme kinetics is vital for the characterization of enzyme targets; furthermore, it is crucial to aid in the design and prosecution of assays to effectively profile compound properties and to gain insight into the mechanism of action required for drug efficacy.
-
Enzyme assays are used extensively in hit identification and hit validation and for detailed characterization of the compound mechanism to guide lead optimization.
-
It is important to understand the limitation of IC50 values and to more deeply probe the relationship between the molecular structures of hits and leads and their kinetics of binding, inhibition and mechanism of action.
-
Combining information from enzyme kinetic studies with that derived from biophysical methods can be advantageous in assessing protein quality, generating suitable assays to identify a range of desired mechanisms and to complement detailed mechanistic characterization.
-
The efficient use of these methods enables the identification, prioritization and progression of truly differentiated compound series that have an enhanced probability of success in translation through to the clinic as a consequence of detailed understanding of their mechanism.
Abstract
Given the therapeutic and commercial success of small-molecule enzyme inhibitors, as exemplified by kinase inhibitors in oncology, a major focus of current drug-discovery and development efforts is on enzyme targets. Understanding the course of an enzyme-catalysed reaction can help to conceptualize different types of inhibitor and to inform the design of screens to identify desired mechanisms. Exploiting this information allows the thorough evaluation of diverse compounds, providing the knowledge required to efficiently optimize leads towards differentiated candidate drugs. This review highlights the rationale for conducting high-quality mechanistic enzymology studies and considers the added value in combining such studies with orthogonal biophysical methods.
Main
Enzyme inhibitors and inactivators comprise roughly half of all marketed drugs1,2 and have transformed human medicine. For example, angiotensin-converting enzyme (ACE) inhibitors3, including captopril4,5 and lysinopril6, which emerged in the late 1970s, now constitute perhaps the most important class of drugs for the treatment of hypertension. ACE inhibitors arose from rational design based on the mechanism of action (MoA) of this metalloproteinase. In addition, in the 1980s, statins, exemplified by atorvastatin7 and lovastatin8, emerged as natural product-derived inhibitors of 3-hydroxy-3-glutaryl CoA reductase and are now the most commonly used drugs to treat hypercholesterolaemia9. Beginning in the 1990s, saquinivir10 and indinavir11 were identified as rationally designed inhibitors of the aspartic protease of HIV-1 (Ref. 12). HIV-1 protease inhibitors have greatly contributed to the conversion of HIV/AIDS from a 'death sentence' to a manageable disease. Furthermore, in the past 20 years, ATP-competitive inhibitors of protein kinases have provided an arsenal of anticancer and anti-inflammatory drugs.
Unsurprisingly, enzymes now typically comprise one-third or more of the discrete drug targets found within the portfolios of large pharmaceutical companies. This is true even for those pharmaceutical companies that have increased their reliance on biopharmaceutical therapies. Because biopharmaceutical agents are generally confined in their utility to extracellular drug targets, intracellular enzymes still may prove to be a growth area for drug discovery.
By their very nature, enzymes are dynamic, with a single enzyme representing a number of different targets as a consequence of binding substrates, intermediates and products during the catalytic cycle. Robertson13 provided a useful compendium of enzymes that are the targets of marketed drugs and makes the case that unlike drug targets of other protein classes, knowledge of the chemical mechanism of an individual enzyme, including the structural characterization of its transition state14,15,16,17, the exploitation of nucleophilic, active-site residues to form covalent adducts with electrophilic inactivators18,19 or the chemical conflation of two enzyme substrates into a bi-substrate analogue20,21, affords the opportunity to design de novo enzyme inhibitors based on the chemical species that emerge during catalysis. Drugs have therefore been designed that target a number of different enzyme forms via covalent and noncovalent inhibition (Fig. 1).
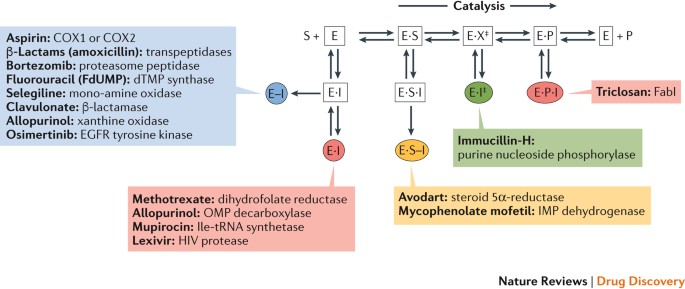
A single enzyme (E) may represent a number of different targets that may be the focus of chemical intervention to modulate activity. By their very nature, enzymes are dynamic and bind substrates (S), intermediates (X) and products (P) during the catalytic cycle. Enzyme inhibitors (I) may bind to these different species either covalently or non-covalently. It is possible to modulate the abundance of these different enzyme species by changing assay conditions to obtain a balance between enzyme forms (to maximize the chances of finding any type of inhibitory mechanism) or to increase the relative concentration of some forms (in order to focus on particular mechanisms). Here, we show a range of different drug molecules eliciting their effects by binding to different enzyme forms via non-covalent (E·I) or covalent (E–I) binding. ‡represents the enzyme's transition state. An early understanding of the enzyme mechanism is advantageous for the identification and subsequent characterization of hits and leads. COX, cyclooxygenase; dTMP, deoxythymidine monophosphate; EGFR, epidermal growth factor receptor; FabI, enoyl-acyl carrier protein reductase; IMP, inosine-5′-monophosphate; OMP, orotidine 5′-phosphate.
PowerPoint slide
Full size image
Understanding the role of enzymes in disease states and the implementation of strategies to modulate their activities for therapeutic benefit remains a key focus for drug discovery. However, while enzymology is a powerful and established discipline, it often fails to receive recognition in providing scientific insight into problems experienced along the route from target identification to proof of concept in the clinic. This is particularly true at the onset of a drug discovery campaign that targets an enzyme. It is uncommon to have a detailed understanding of the kinetic mechanism (the order of substrate addition and product release) and the chemical mechanism of catalysis of an enzyme target before the initiation of 'hit' discovery. Ironically, at this early stage of a programme, an X-ray structure of the enzyme target may be extant even in the absence of an understanding of its detailed mechanism of catalysis. As a result, the opportunity for the use of rational design to afford enzyme inhibitors or inactivators based on the enzyme mechanism may have been eclipsed by a medicinal chemistry effort that focuses on a limited number of chemotypes that in no way resemble the substrates of the enzyme target. Additionally, following the knowledge gleaned from more than 20 years of 'random' high-throughput screening (HTS) applied to hundreds of enzyme targets, pharmaceutical companies are increasingly capable of predicting which classes of enzyme targets in their active portfolios will provide durable lead compounds from HTS campaigns and which are likely to fail to do so.
For these and other reasons, mechanistic studies of enzyme targets are now enjoying a renaissance, with many pharmaceutical companies increasing their focus on using detailed, more physiologically relevant enzyme kinetic studies during the lead identification and optimization phases as another measure to address the increasingly high cost and low success rates of drug discovery within the industry. There is renewed recognition that (mechanistic) enzymology is essential for establishing robust, reliable and appropriate assays for screening campaigns to identify hit compounds that are also adaptable to comprehensively characterize inhibitors more fully than traditional half-maximal inhibitory concentration (IC50) values alone. While useful, the information inherent in IC50 values does not indicate a change in inhibitory mechanism (for example, a shift from competitive to mixed-type inhibition) in a series of lead compounds and moreover, the data are poorly correlative with their pharmacodynamic action, as they give no measure of the period of time the compound 'resides' on its target22.
The purpose of these MoA studies is to characterize the interaction of a compound with its target and to understand how natural ligands at physiological concentrations will modulate this activity. Ideally, data from a series of hit compounds are annotated in terms of potency, selectivity versus homologous targets and their developability properties, and MoA data are delivered for all lead and drug candidates. This practice provides a full 'data dossier' for the advancement of lead compounds to clinical candidates with a well-characterized MoA, cellular efficacy and the appropriate safety and pharmacokinetic properties. This is particularly true when one considers the difficulty of registering a drug that has no known functional target23. Additionally, it allows the identification and prioritization of not just a single mechanism but potentially a range of diverse mechanisms, thoroughly characterized using increasingly complex assays (isolated protein through to tissues), to produce a number of differentiated options to achieve the desired biological effect in vivo.
Of course, enzymology alone cannot deliver all of the required data or information needed in early drug discovery, and its combination with biophysical methods and protein structural analysis remains essential for providing an integrated pharmacological view of the thermodynamics and kinetics of enzyme catalysis and inhibition24. Furthermore, integrating the understanding derived from detailed mechanistic characterization in isolated enzyme assays coupled with cell-based assays and, ultimately, with metabolic pathways and systems knowledge will continue to identify enzymology as one of the most important quantitative disciplines within drug discovery.
This article will discuss the importance of conducting high-quality mechanistic enzymology studies, consider how detailed and early knowledge of an enzyme mechanism may add value throughout a drug-discovery programme and assess the value of combining such knowledge with orthogonal biophysical methods.
Pre-screening considerations
What is the appropriate biochemical nature of an enzyme target and its substrate? When a decision is made to pursue a discrete enzyme as a drug target, the optimal biochemical nature of that enzyme and its substrates becomes the subject of debate. Does one insist on a purely cellular environment for the enzyme and its substrates to maintain biological integrity or is one able to proceed with a purified recombinant enzyme and substrates that may not resemble its native substrates? As one example, the development of HIV-1 protease inhibitors as drugs was achieved with a recombinant decrement of the protease and with using non-protein, oligopeptide substrates resembling cognate cleavage sites in retroviral polyproteins15. However, as elaborated below, protein kinases provide interesting examples in which a recombinant 'biochemical' enzyme species must more closely conform to its native 'biological' entity25.
Classical drug-discovery campaigns generally include a combination of biochemical, biophysical and cell biology assays and methodologies to identify and characterize modulators of key targets and pathways of interest. In order to derive the most relevant insights from the various approaches with the goal of translating in vitro data to a clinical disease setting, a number of important considerations are required.
Physiological environment
A comprehensive understanding of the molecular environment of the target of interest is crucial to enable the design of more physiologically relevant assays. Recent times have seen the pharmaceutical industry moving away from the use of isolated, usually recombinant enzyme domains, model or generic substrates and returning towards a focus on systems likely to be of higher relevance and translatability. This has resulted in a greater number of assays employing full-length enzymes, complex partners and native substrates, requiring a more detailed analysis of the underlying enzymology.
It is important to consider that in the complex physiological environment, full-length, multi-domain enzymes comprise catalytic domains that can be regulated by non-catalytic domains in a manner that controls activity, substrate specificity and potentially inhibitor structure–activity relationships (SARs). The AKT serine/threonine protein kinases (AKT1–3) provide an example. A research strategy that utilized a recombinant fragment encompassing only the catalytic domain of this membrane-associated protein kinase, rather than the native, full-length protein, would have overlooked the allosteric site on the amino-terminal pleckstrin homology domain that ultimately provided an exploitable site for inhibitor discovery26. While one pharmaceutical company could have decided that recombinant expression of the kinase domain of the AKT kinases would prove more expeditious and more amenable to structural characterization, Merck chose the path of producing the 'native' kinase (including the pleckstrin homology domain) and thereby discovered a new site for enzyme inhibition, defining a new chemical equity26,27 (Table 1). In another example, a kinetic analysis using tyrosine protein kinase ITK/TSK (ITK) as a model system to understand the regulation of TEC family kinases, identified a 17-residue linker sequence between the SH2 and kinase domains as a critical component that maintains ITK kinase activity, along with several specific residues that mediate interactions between this regulatory region and the catalytic domain of ITK28. In this system, the use of the isolated kinase domain would be poorly representative of the kinetic characteristics of the enzyme. Furthermore, demonstrating also the impact of protein tags, the k cat value for the hexahistidine-tagged cytoplasmic domain of hepatocyte growth factor receptor (HGFR; also known as c-MET) has been reported to be 0.0095 s−1 (Ref. 29), whereas the k cat value for the untagged catalytic domain has been reported to be 1 s−1 (unphosphorylated c-MET) and 31 s−1 (autophosphorylated c-MET)30.
Full size table
With respect to small-molecule inhibitors, U0126 has been reported to inhibit the truncated, constitutively active recombinant kinase domain of dual specificity mitogen-activated protein kinase (MAPK) kinase 1 (MKK1) with an IC50 of ~70 nM, whereas it inhibits the full-length endogenously activated wild-type enzyme isolated from cells with an IC50 of ~500 nM (Ref. 31). In an even more striking example, the addition of a 19-amino acid juxta membrane domain to the catalytic domain of vascular endothelial growth factor receptor (VEGFR) increases the affinity of a small-molecule inhibitor from 1,100 pM to 28 pM (Ref. 32). In another example, the catalytic domain of the human β-amyloid precursor protein cleaving enzyme (BACE1) was reported to essentially function independently of the rest of the protein and hence can be used as a surrogate for the full-length protein33.
Detailed characterization and comparison of the mechanism and key kinetic parameters between the different enzyme forms are required in order to be confident of the relevance of the isolated domain with regard to the pathophysiological state of the enzyme. Domain architecture, post-translational modification status, activation state and artificial modifications such as affinity tags can all affect the kinetic integrity of the enzyme.
Binding partners
Complex binding partners can also exert significant influence on the catalytic activity of the associated enzyme, a classic example being the cyclin partners of cyclin-dependent kinase (CDK) enzymes, where activation of the cyclin-dependent kinases is a two-step process comprising cyclin binding, followed by phosphorylation at a conserved threonine residue within the kinase activation loop34. Such modifications have the potential to also affect inhibitor binding. For example, targeting protein for XKLP2 (TPX2) is a physiological binding partner and positive regulator of Aurora A kinase (AurA); while the presence of TPX2 has no effect on the reaction mechanism and enzyme turnover, it does affect the binding affinity of the kinase's substrates and also the SAR of inhibitors binding to a hydrophobic pocket adjacent to the ATP binding site35 (Table 1). Profiling in the presence of the native binding partner can clearly enhance the probability of identifying molecules that are more likely to have an impact in physiological environments. More than a single enzyme subunit may come into play. For example, the histone-lysine N-methyltransferase EZH2 can fully catalyse the methylation of histone H3 lysine 27 only in the presence of its associated partners comprising its full pentameric Polycomb repressive complex 236. Hence, an understanding of the physiologically relevant protein complex is key to ensuring that the correct form of the enzyme is represented. Indeed, there may be specific complexes associated with health-relevant and disease-relevant states of the enzyme, the knowledge of which can help to drive selectivity. A study at Agios Pharmaceuticals used metabolomics to discover that 2-hydroxyglutarate, an agent that causes malignant gliomas, results from the neomorphic activity of human isocitrate dehydrogenase (IDH1), which, owing to a single point mutation (R132H), catalyses its formation from α-ketoglutarate37. Immunoprecipitation from disease-relevant cells coupled with mass spectrometry analysis can help to identify complex partners and their associated stoichiometry38.
Enzyme substrates
The biochemical nature, or identity, of enzyme substrates is another important consideration. There are numerous examples where binding interactions between macromolecular substrates and enzymes occur at sites distal to the active site and indeed can contribute to the overall binding energy for the formation of the initial enzyme–substrate (ES) encounter complex39. Substitution of the native substrate with, for example, in the case of a protease or kinase, a shorter peptide could affect both the kinetic mechanism of the enzyme and the ability to identify inhibitors that might prevent formation of the ES complex by binding to distal sites, particularly if specific conformational changes accompany binding of the native substrate. For example, specific inhibitor pockets are revealed upon substrate binding in bacterial Glu-tRNAGln amidotransferases40. Among protein kinases are numerous examples in which the kinetic mechanism and small-molecule affinity are directly influenced by the choice of substrate. When MAPK p38α catalyses phosphoryl transfer to the cAMP-dependent transcription factor ATF2, the data support a rapid-equilibrium, random-order mechanism of substrate addition41 (or ordered with ATF2 protein binding first42). However, in the presence of a short peptide as a phosphoacceptor, the reaction proceeds through an ordered mechanism with MgATP binding first43. A similar scenario is recognized for 3-phophoinositide-dependent protein kinase 1 (PDK1), in which phosphorylation of an extended peptide substrate containing a single distal recognition element reacts through a rapid-equilibrium, random-order mechanism44. However, phosphorylation of the native downstream substrate S6K1 protein kinase occurs through a steady-state ordered mechanism in which binding of S6K1 precludes association of MgATP45. Detailed understanding of the catalytic mechanism and full consideration of the various enzyme–substrate interactions can afford control over the specific downstream pathways to be inhibited in a cellular context. For example, in the case of p38, differential substrate selectivity is able to define the particular pathways affected by small molecules, offering exquisite control and, potentially, fewer unwanted side effects46,47 (Table 1).
For a number of years in the epigenetic field, the importance of using native nucleosome substrates in preference to short, histone N-terminal tail peptides as surrogates has been realized in order to identify small-molecule inhibitors that translate through to the cellular context34 and target the desired activity of the enzyme. In the case of the histone-lysine N-methyltransferase NSD2, for example, the specificity of lysine methylation is directed by the choice of a nucleosome substrate versus a histone octamer substrate in the presence of short single-stranded DNA or double-stranded DNA48. In order to elucidate the true structure of the transition states of the NSD2-catalysed methylation of Lys36 of histone H3, methylated, full-length nucleosomes were required as substrates to provide fidelity of the biological target in a panel of kinetic isotope effect studies49. This reiterates an important observation that while many enzymes can be observed to catalyse low-level activity using surrogate substrates, the physiological relevance of this needs to be verified50. These examples illustrate the importance of understanding the most physiologically relevant form of the enzyme to study and, indeed, target. Moreover, if it is not always feasible to perform high-throughput hit-finding campaigns with full-length protein and native substrates, it is crucial to explore the level of compromise imbued by the use of simpler systems and define a strategy to relate any activities back to the cellular context.
A clear advantage of having detailed knowledge of the molecular environment of the enzyme in the disease-relevant setting is the ability to prioritize specific mechanisms of interest to target. For example, it may be preferable to identify inhibitors that are not competitive with the native substrate where the cellular concentration of the latter is high; an emerging focus in the field of kinase drug discovery is on the identification of inhibitors that are either non-competitive inhibitors or uncompetitive inhibitors with respect to ATP, in order to circumvent the challenge of high cellular concentrations of ATP51. While rare, enzyme inhibitors that operate by uncompetitive inhibition are ideal for disrupting enzyme targets within metabolic pathways because the resulting accumulation of the substrate cannot thwart inhibition as it is not competitive52.
An example of an uncompetitive inhibitor is the natural product mycophenolic acid, which targets inosine 5′-monophosphate dehydrogenase (IMPDH). IMPDH catalyses hydride transfer from C2 of IMP to the co-substrate NAD+, and XMP is produced by addition of water to IMP. The rate-limiting step in the mechanism of mammalian IMPDH is the hydrolysis of a covalent thioimidate complex formed between the nascent XMP product and an active-site cysteine. Mycophenolate binds to this IMPDH–IMP covalent complex to exploit this slow step in catalysis53.
Enzyme kinetics
Is an understanding of the rate-limiting step of a target enzyme before the launch of a drug discovery programme essential? The rates of the majority of enzyme-catalysed reactions are limited by either chemical or product release steps. In the latter case, an enzyme–product complex will be the predominant enzyme form under steady-state conditions, especially at high concentrations of substrate. One could envision a compound screening strategy wherein an enzyme–product complex or a mimic thereof is enriched in the assay conditions. In this way, HTS would survey an enzyme species with a partially blocked active site, such that a 'hit' compound, upon binding to a discrete subsite, would inhibit the enzyme by preventing desorption of the product ligand. Such an inhibitor would generally effect uncompetitive inhibition of the target enzyme. Importantly, screening an enzyme–product complex samples a different inhibitor space than the free enzyme, and the formation of an inhibited ternary complex may provide higher selectivity than simply binding to the free enzyme. A single plate in such an HTS campaign could be screened twice, once with no added product analogue, and once with such an analogue. The first plate would reveal competitive or mixed-type inhibitors, while the latter would preferentially uncover uncompetitive inhibitors. For example, the rate-limiting step of the bacterial enoyl reductases encoded by the FABI and INHA genes is the release of the product NAD+ (Ref. 54). If one were to conduct HTS in the presence and absence of added NAD+ or its analogue, such as acetyl-pyridine adenine dinucleotide, hit compounds that bind more tightly to the enzyme–nicotinamide complex rather than the free enzyme might provide a more attractive series of hit compounds to lead optimization.
Consideration of the rates of binding and desorption, k on and k off, can also be key in designing inhibitors that exhibit kinetic selectivity in which the drug–target complex has a longer half-life than off-target–drug complexes. Slow-binding inhibition kinetics are a feature of many marketed drugs (as summarized in Ref. 55), and indeed, the concept of residence time has been an important consideration in the translation of such kinetics into cellular and in vivo contexts22,56. A prospective mechanistic pharmacodynamic model to predict dose–response curves for inhibitors of the LpxC enzyme from Pseudomonas aeruginosa in an animal model of infection, encompassing drug–target kinetic parameters, including the on and off rates for the formation and breakdown of the drug–target complex, has recently been described57. Irreversible covalent inhibition is also gaining attention, where tuning the balance between the initial molecular recognition event and the chemical reactivity can lead to the desired target selectivity without compromising safety and concern for off-target toxicity19,58.
Screening assays
Screening assays can be built in a number of ways. As mentioned above, they can be devised to identify inhibitors with a specific mechanism (for example, the substrate-uncompetitive mechanism by ensuring the majority of the enzyme species sampled in the assay exists in the ES form) or to target the rate-limiting step of catalysis. Assays can be balanced to ensure that a holistic breadth of modes of inhibition is captured (for example, by ensuring that the population of free enzyme E is equivalent to the population of the ES complex under conditions in which the concentration of substrate equates to the Michaelis constant (K m))59. They can also discriminate against unwanted mechanisms (for example, bias away from competitive inhibition by ensuring an excess of substrate is present in the reaction). This ability to control the transitory species of enzymes can exist only as a consequence of a detailed understanding of the kinetic mechanism in relation to the physiological substrate conditions. This is particularly important for multisubstrate enzyme reactions, where a comprehensive dissection of the mechanism and understanding of the relative proportions of enzyme species existing at any point during the reaction require determination of the kinetic mechanism, order of substrate addition and product release and apparent substrate cooperativity; this is achieved through a combination of product and dead-end inhibition studies, kinetic isotope effects and, where relevant, identification of covalent reaction intermediates. A detailed description is beyond the scope of this review; however, interested readers are directed to Segal60 for further description and to Schneck et al.61 for a comprehensive analysis of cathepsin C using such studies. A sophisticated example showing detailed analysis of four enzymes in the shikimate pathway (Aro B, D, E and K) of Streptococcus pneumoniae illustrates how such studies enable balanced assay conditions to be generated62. Similarly, elegant kinetic studies of focal adhesion kinase 1 (FAK1)63 demonstrated that drug discovery efforts for this or kinetically similar protein kinases could focus on developing inhibitors that trap the enzyme in the 'locked-down' ATP-bound or ADP-bound closed form. Clearly, an understanding of the molecular enzymology of our drug discovery targets enables fine tuning of the assays in an intelligent and sophisticated manner to enable optimization of mechanistically novel chemical equity that will translate to be efficacious against physiologically relevant enzyme species.
Post-screening: understanding IC50
Following the identification of hits, their inhibitory effect must then be quantified, so that chemistry design efforts may be applied to those compounds most likely to have the best chance of progression. Historically, this has been achieved by measuring the concentration at which the compound elicits 50% of its maximal inhibitory effect (IC50). To obtain this value, concentration–response analyses are undertaken, whereby the degree of enzymatic inhibition is monitored at increasing concentrations of the inhibitory test compound. Although these types of experiments are fairly straightforward to perform, it should be remembered that the IC50 itself may not be a true reflection of inhibitor affinity, as the measured value may be significantly affected by experimental conditions, particularly the concentration of the substrate to which it is competitive.
As well as potential differences associated with buffer components, pH and ionic strength effects, binding often involves protein flexibility64 and changes in conformation during induced fit, where the binding site adapts to the shape of the ligand processes. The value of the IC50 may be modified by changes in substrate identity and concentration as well as enzyme concentration. Additionally, in traditional analyses of IC50 values, the enzyme, substrates and inhibitor are co-mingled for a common period of exposure. Any effects of time-dependent inhibition are undetectable in this assay format. An enzyme target inside a cell will experience frequent exposure to its substrates but, owing to pharmacokinetics, only temporary exposure to an added inhibitor. Under these conditions, the IC50 values of the primary enzyme target and its off-target homologues in separate assays receive similar temporal exposure to a panel of inhibitors, regardless of whether these targets are all colocalized in a germane cell. A more useful measure of enzyme inhibition of hit or lead compounds may involve pre-incubation of the enzyme and inhibitor, followed by its dilution, before assessment of residual activity by added substrates. Such an approach would unveil tight-binding or time-dependent inhibitors and possibly demonstrate that while time-dependent inhibition is operative for a primary target, this does not occur with secondary targets. The effects of some commonly observed situations in determining IC50 are shown in Fig. 2.
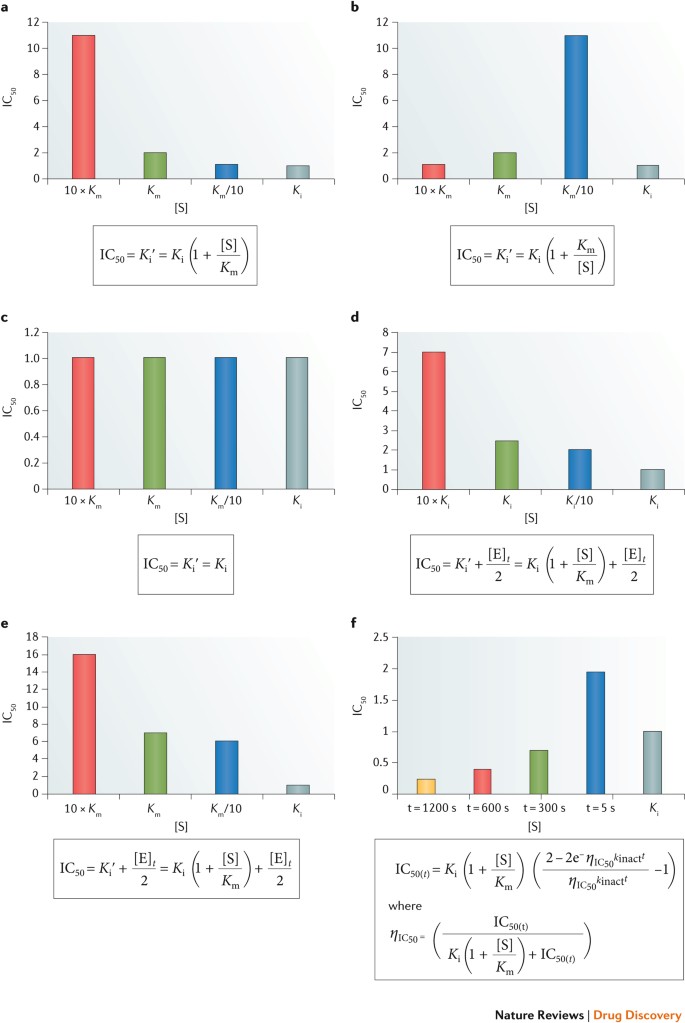
Graphical representation of the effects of changes in assay conditions (substrate concentration [S], enzyme concentration [E] or time t) on the magnitude of the measured half-maximal inhibitory concentration (IC50) for selected types of inhibition. Panels a, b and c show the expected response for standard competitive, uncompetitive and non-competitive inhibition. Panels d and e display the consequences of tight-binding inhibition for the competitive model. Panel f shows the effect of time on the apparent IC50 for an irreversible inhibitor. In all instances, the inhibitor constant K i = 1 nM. The effect of changing substrate concentration is shown for concentrations at the Michaelis constant (K m) and 10-fold above and below K m. Where enzyme concentration is varied, it is shown at K i and 10-fold above and below K i. For the time-dependent effects, time is varied between 5 and 1,200 s.
PowerPoint slide
Full size image
Mechanism of action
Spurious mechanisms. There has been a growing appreciation of the requirement to identify and remove compounds showing activity in primary screens via undesired mechanisms from primary screening hit lists65. Perhaps unsurprisingly, there are many specific mechanisms by which so-called nonspecific inhibition, sometimes known as promiscuous inhibition, can occur. These include common technology hitters50, impurities (such as heavy metals)66, precipitators67, aggregators68, redox active compounds69, intrinsically reactive compounds70 and compounds that bind preferentially to unfolded proteins. Promiscuous compounds effective against protein kinase C (PKC) and MKK1 by virtue of an aggregation affect, which effectively reduces the concentration of available active enzyme, have been identified71 (Table 1).
Collectively, these compounds have been termed pan-assay interference compounds (PAINS)72 and their presence may result in misleading IC50 values. This is because the inhibitory activity is not related to the stoichiometric inhibition of the protein but results from other effects that may lead to inactivation, denaturation or removal of active protein from its usual catalytic or binding function73,74. Several computational approaches75 have been designed to identify such compounds, and suggestions to remove them from screening sets have been made. However, the activity of such compounds is often dependent on both protein and assay composition, and so these approaches may not be accurate or required. This means that enzyme kinetics-based methods applied for a particular protein under relevant assay conditions are the preferred route for annotating such compounds.
There are several key steps that can easily be employed to help to identify PAINS, including assessing the steepness of the slope of the inhibition curve, investigating the time dependency and enzyme concentration-dependency of the observed inhibition, monitoring the effect of increasing detergent concentration, measuring the potential for inhibition in other assays with unrelated enzymes, characterizing the reactivity with reducing agents and monitoring the effects on protein stability and unfolding.
Often PAINS give characteristic responses in these types of experiments, such as steep slopes, as occurs by rottlerin inhibition of β-lactamase76, and time-dependent and/or enzyme-dependent inhibition. Several promiscuous kinase inhibitors were demonstrated to have time-dependent inhibitory effects on a number of unrelated enzymes77. Often, the inhibition may be reduced by adding detergent, and sometimes, these compounds will be active at the same affinity range as unrelated enzymes. The effect on protein stability can be investigated using a modification to Selwyn's test78 or by employing biophysical methods.
Of course, it is essential to position these assays soon after initial hits have been identified, so that compounds potentially displaying these unwanted mechanisms can be assessed, annotated and prioritized accordingly. It should be noted that this does not mean that compounds possessing mechanisms such as those listed above are automatically ruled out from further optimization. Sometimes the promiscuous activity occurs only at concentrations much greater than those that are required for true, specific, binding-based inhibition, and in this case, compounds can move forward in the drug-discovery process. The important factor is that an understanding of the potential liabilities is generated early, so that an informed decision-making process can take place.
Slow-binding and tight-binding enzyme inhibition. Enzyme inhibitors can act either reversibly or irreversibly, and the basic principles are well-described in a number of classical enzymology textbooks79. The time-dependent properties of enzyme inhibitors constitute a continuum, wherein some compounds that covalently inactivate an enzyme actually demonstrate more reversibility than a noncovalent inhibitor that achieves a remarkably tight binary complex. These modes of inhibition show very different behaviour both in vitro and in vivo 80. However, reversibility is not an absolute phenomenon, since inhibition considered to be irreversible may actually be reversible over a period of time that is much greater than the usual assay time. This leads to an operational definition that classifies inhibition as irreversible if the loss of enzyme activity caused by an inhibitor is not restorable over the timescale of the enzyme activity assay.
For reversible inhibition, full inhibition is usually obtained rapidly because there is no chemical reaction involved, only a simple noncovalent interaction. It should be noted, however, that irreversible inhibitors are not required to form covalent bonds and that there may be covalent bond formation between a reversible inhibitor and the enzyme. The distinction between reversible and irreversible inhibitors is made only on kinetic grounds. Compounds that fall in the continuum of reversible and irreversible inhibition are known as slow-binding inhibitors57, and slow-binding inhibition, which usually accompanies tight-binding inhibition, is a widely occurring phenomenon during drug discovery13. This nomenclature may, however, be misleading, as many of these compounds associate rapidly with the enzyme and then slowly isomerize into a higher affinity form — for example, this is the mechanism responsible for the isoniazid–NAD adduct inhibition of INHA81 and is also observed for some metal binding inhibitors of HIV-1 integrase82. Dissociation of the inhibitor is also slow. Hence, for a slow-binding inhibitor, on rates can be fast or slow, but off rates are always slow. Slow binding is operationally defined as an increase in inhibition during the time of the assay. Irreversible inhibition can be considered a special type of slow binding in which recovery of the enzyme activity is too slow to detect.
Slow-binding inhibition. Because the degree of inhibition for a slow-binding inhibitor increases with time, the IC50 also changes with time, and so an IC50 value obtained at a single time point may not be a reliable measure of inhibitory potency.
It is crucial to recognize the importance of analysing time courses for inhibitors suspected to be slow binding or irreversible, rather than using single time points for dose–response analysis, as failure to do this could result in the misannotation of potency and misleading information as to the MoA. Instead, it is necessary to characterize the rate constants of the steps involved in forming the enzyme–inhibitor (EI) complexes. An example of such characterization is that of extracellular signal-regulated kinase 1 (ERK1; also known as MAPK3) and ERK2, in which both slow association and dissociation of Vertex-11e and SCH772984 were observed, with apparent k on values of 0.21 and 2.8 μM−1 s−1, respectively, and dissociation rate constants of 0.21 and 1.1 h−1, respectively83 (Table 1).
There are two important, distinct mechanisms that account for the majority of slow-binding interactions84. The first occurs because, according to the law of mass action, reactions at low concentrations will proceed more slowly. When an inhibitor has a low inhibition constant (K i) and the inhibitor concentration [I] is varied in the region of K i, the values of the pseudo-first-order rate constant for the formation of the non-covalent enzyme–inhibitor (E·I) complex (k 3 × [I] t ) and the dissociation rate constant (k 4) would be low. Slow-binding inhibition may also occur because of a low value of k 3, and such a mechanism is represented in Fig. 3 (mechanism A). These low values of association and dissociation would lead to slow binding even though k 3 may be of the order expected for a diffusion controlled reaction (>108 M−1 s−1). It is the product of the true second-order rate constant (k 3), [I] t and the enzyme concentration [E] that determines the rate of E·I complex formation. It can also be seen that if [E] is very low, possibly because the majority of [E] t is actually in the form ES due to the presence of a very highly competing substrate, slow binding may result.
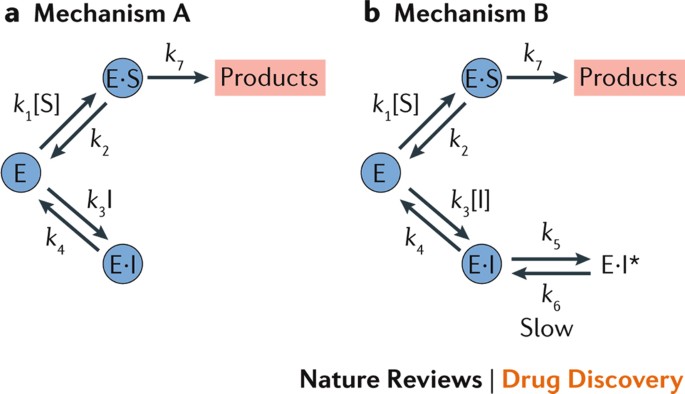
Two potential mechanisms have been proposed to describe the observation of slow-binding enzyme inhibition. These are shown in parts a and b. Part a illustrates the single-step slow-binding mechanism, in which the formation of the non-covalent enzyme–inhibitor (E·I) complex is slow, and part b illustrates the two-step slow-binding mechanism, in which E·I is formed rapidly but is then slowly converted to the higher affinity E·I* complex. E·S, non-covalent enzyme–substrate complex; [I], inhibitor concentration; k 1–7, rate constant of the specific step; [S], substrate concentration.
PowerPoint slide
Full size image
Slow-binding inhibition can also arise from the rapid formation of an initial collision complex (E·I), which subsequently undergoes slow isomerization to a higher affinity complex (E·I*). A scheme for this type of slow-binding inhibition, often termed mechanism B, is shown in Fig. 3 (mechanism B).
It has been suggested that the majority of slow-binding and slow, tight-binding inhibitions occur according to mechanism B85. It is envisaged that inhibitors initially combine with enzymes at their active sites and subsequently induce conformational changes that cause an increase in affinity and the formation of a more stable EI complex, from which inhibitor is slowly released.
Hence, for inhibition to conform to mechanism B, K i* must be lower than K i, and therefore, k 6 must be lower than k 5. In addition, the values of k 5 and k 6 must be of a magnitude that allows observation of the attainment of equilibrium between E·I and E·I*.
By following the progress curves for inhibition, in reactions starting with enzyme, it is possible to distinguish between these two different mechanisms of slow-binding inhibition using nonlinear regression analysis aided by secondary plots of k obs versus [I], which are linear for the one-step mechanism and hyperbolic for the two-step mechanism, and to estimate the individual rate constants.
Another useful approach is to carry out jump-dilution experiments, where the pre-equilibrated E·I complex is diluted rapidly into the assay and recovery of enzyme activity is monitored as the inhibitor dissociates from the complex86. These experiments are aided by the use of high substrate concentrations, which may serve to compete with the inhibitor for rebinding to the free enzyme and to increase the detectable signal.
Tight-binding inhibition. The drug-discovery process, by its very nature, often results in the identification of high-affinity molecules. Owing to this high affinity, these compounds are often difficult to characterize. In order to rank compounds correctly, it is important to realize that true K i values must be compared and that IC50 values do not give an accurate representation of affinity under certain conditions, for example, situations in which ligand depletion occurs87. This situation is often termed 'tight binding', although it really relates to a situation where the active enzyme concentration is approximately equal to, or even higher than, the apparent inhibition constant. This means that tight binding could occur even for weaker compounds at elevated enzyme concentrations. This phenomenon has been known for many years through the relationship among IC50, K i′ (the apparent K i) and [E] t described by Goldstein88 in 1944 (equation 1):
where K i′ = K i/(1 + [S]/K m). It is clear from equation 1 that the measured IC50 is limited to half of the functional enzyme concentration as the K i′ value becomes very small and, conversely, that the IC50 approaches the apparent K i value only when the functional enzyme concentration is small relative to the apparent K i value. The consequence for drug discovery is profound, IC50 cannot be used to rank compounds when the affinity approaches the enzyme concentration. When this situation occurs, additional approaches must be taken to fully understand the true affinity.
Although there are data-fitting approaches that do not assume that the free concentration of inhibitor equals the total concentration, such as the quadratic equation described by Morrison89 in equation 2, even these methods become unreliable as the affinity increases beyond around 10-fold below the functional enzyme concentration.
where v i is the inhibited rate, v 0 is the uninhibited rate and [I] t is the total inhibitor concentration. When [I]t = IC50, v i = 0.5v 0, and equation 2 can be rearranged to provide equation 1.
Experimental approaches to decrease the functional enzyme concentration or raise the apparent K i by the use of increased concentrations of competing substrate may have limited utility, as assays are often initially configured with low concentrations of enzyme to avoid this issue; therefore, further decreases can cause issues with the biosignal sensitivity. Shifting K i′ is possible only for compounds that have a competitive component to binding, and often limited shifts may be possible due to limited solubility restricting the achievable concentration:K m ratio. Experimental conditions for accurate determination of K i values for tight-binding enzyme inhibitors, following an in silico study of experimental error and assay design, has been described90. In practice, it may mean that biophysical and/or kinetic- based methods need to be used to quantify the dissociation constant (K d), and efforts need to be made to relate the constant to K i.
Different enzyme forms
Enzyme kinetic assays must, by definition, sample a number of different enzyme species during the catalytic cycle, as substrates are bound, intermediates are formed and products are released during the enzyme-catalysed reaction, and inhibitors may potentially access a number of these forms91 (Fig. 1). Additionally, other enzyme forms, such as different post-translational modifications, redox states or partial degradation products may also be present even if the experimenter is unaware of their presence (although efforts should be made to identify such additional enzyme forms). These potential additional forms may have varying catalytic efficiencies and sensitivities to the test compound and thus may contribute to the measured IC50 in undefined ways. It is therefore prudent to undertake rigorous quality control assessments as detailed in order to understand the contribution of different enzyme forms to the total enzyme concentration and the total catalytic rate, so that the magnitude of this issue can be estimated. It is also important to repeat these assessments on new batches of protein to ensure that the degree of any changes in the amount of modified enzyme forms is known.
Irreversible covalent compounds
Irreversible, covalent compounds19,92 are, by definition, unable to be ranked in terms of effectiveness using an equilibrium dissociation constant, K d, or K i. This means that IC50 values are also not useful in ranking these types of compounds. This should be obvious, as any irreversible inhibitor added at half the enzyme concentration will, given long enough for all of the added inhibitor to covalently modify the enzyme, lead to a 50% decrease in the control rate. This is the extreme case in equation 1, where K i′ = 0 and so IC50 = [E] t /2. However, it is still possible to carry out concentration responses with irreversible compounds under fixed conditions of substrate concentration and time, although this is not recommended to assess irreversible inhibitor effectiveness. Instead, this should be assessed using the kinetic parameter k inact/K i, which is ascertained from time-course experiments with varying inhibitor concentrations93, where the rate constant, k obs, represents the conversion from the initial complex to the irreversible covalent complex. Here, k inact is the rate constant for inactivation, while the K i term describes the concentration of inhibitor required for one-half of the maximum rate of covalent bond formation and should not be confused with K i describing the dissociation of the E·I complex, which is not affected by covalent bond formation. For a 2-step reaction, k inact represents the maximum observed rate constant for inactivation at saturating [I].
Attempts have also been made to derive these parameters from time-dependent IC50 values94. The advantage of using a kinetic approach to measure k inact/K i is two-fold: it uses valid parameters to describe the inhibition, and it allows the effectiveness of the irreversible inhibition to be dissected into initial molecular recognition and chemical reaction steps under the conditions of mechanism B (Scheme 2), from which k inact/K i and k inact can be ascertained. The ability to characterize irreversible inhibitors in this way is essential to modulate the balance between intrinsic compound reactivity, which may lead to a lack of selectivity, and initial noncovalent interactions, which help improve recognition of the target protein95,96. Table 1 also illustrates the dissection of the irreversible inhibition of epidermal growth factor receptor (EGFR) tyrosine kinase97. Thus, having these key parameters available for lead series during a lead optimization campaign allows the medicinal chemist to tailor the requirements of initial affinity and reactivity in order to mitigate concerns over reactive warheads and potential off-target toxicity.
Mechanism of inhibition
Test compounds may have a number of different mechanisms with respect to the effects of substrates or, indeed, other ligands on the degree of inhibition98. Competitive inhibition is common, as inhibitors are often either designed to mimic the substrates of an enzyme reaction or to bind at the substrate-binding site, frequently resulting in the observation of competitive kinetics. Other mechanisms, however, are also possible, including non-competitive kinetics, where a test compound may bind both before and after substrate binding (and in the case in which the affinities for the free enzyme and ES complex are different, the inhibition is classified as mixed inhibition). As mentioned above, uncompetitive inhibition is rarer and occurs when the inhibitor binds only after the substrate has bound to the enzyme, such as the case in which mycophenolic acid binds to an inosine 5′-monophosphate dehydrogenase that is covalently complexed to its nucleotide substrate52,99,100.
Once inhibitors have been identified during primary screening, it is important to understand how the concentration of substrates affects the measured IC50 values, as this allows the mechanism of inhibition to be determined and provides a basis for extrapolating the activity seen in isolated protein assays to effects observed in cells, where the concentration of substrates may be different to the biochemical enzyme assay. It also allows for effective assessment of selectivity, as the magnitude and direction of inhibitor selectivity can vary according to the mechanism of inhibition and the substrate concentration at which selectivity is assessed. These observations are well described in a number of classical enzymology texts101.
Initial experiments to determine the mechanism are often focused on two substrate concentrations, above and below the K m of the varied substrate. While this may give an indication of the mechanism of inhibition, it is recommended that full matrix-based experiments covering a range of substrate and inhibitor concentrations are undertaken, rather than this sparse data approach, so that reliable K i values can be estimated.
Of course, it is also important to use a relevant modified form of the Cheng–Prusoff equation to ensure that the estimated parameter values reflect the nature of the assay system102 and to investigate instances of cooperative binding, which may also require more complex equations when the slope does not equal 1 (Ref. 103).
Data analysis
Concomitant with the issues described above, misleading IC50 values may also arise via improper data analysis104. Although many software packages are available and are used routinely to apply nonlinear regression analysis to calculate parameter values, it is often difficult to know whether an appropriate concentration–response equation has been fitted to the data. For example, in many companies, four-parameter logistic equations or variations thereof are automatically used to analyse HTS concentration–response data, even if the system is adequately described by a simpler equation or should be described by an alternative, more complex equation. Of course, the equation used should ideally directly relate to the physical processes occurring in the assay to allow meaningful estimates of relevant parameters, such as inhibition of a specific enzyme species. Additionally, the experimenter should ideally assess and record whether the equation provides an adequate quality of fit, generates precise and accurate parameter values and highlights any redundant parameters105. Typically, and certainly historically, this is not possible during the concentration–response analysis of several thousand compounds, and so the reality is that many company databases may contain large numbers of IC50 values that do not correspond to the true potency of the test compound.
Combining enzymology and biophysics
As we have seen above, mechanistic enzymology is a powerful approach, which when applied carefully, can allow for extensive characterization of protein–modulator activity.
However, combining or supplementing enzyme kinetic studies with biophysical methods can add value at many stages in the drug discovery process (Table 2). Since the mid-1990s, biophysical methods have been increasingly used in this way, and there are now many methods106 that can be routinely applied in a number of settings to enhance the understanding of the molecular MoA of test compounds.
Full size table
This combination extends through initial reagent characterization (including protein and tool compound evaluations), assay development and choice of HTS conditions to more detailed MoA studies during lead optimization (Table 2).
Combining these highly informative studies early in each of these phases has the advantage of increased impact beyond that delivered by each alone and crucially allows for cost savings to be realized due to unsuitable reagents, incorrect assumptions regarding tool molecules and inappropriate assay configuration to detect desired MoAs. A combination of biophysical methods to interrogate binding kinetics and protein integrity, coupled with detailed dissection of the enzyme catalytic mechanism and effects of intervention by small-molecule modulators, provides a comprehensive level of detail at the molecular level. This interplay is impactful at all stages of target (+/− ligand) characterization.
Combining catalytic and binding assays
Reagent evaluation. Before target proteins are used for assays, there are several important quality control measures that should be conducted. The identity, purity, concentration, functionality and stability of the protein must be confirmed. Biophysical methods may be used to impact all of these areas, but consideration alongside the enzyme kinetic data provides crucial context-dependent information.
Amino-acid analysis (AAA), liquid chromatography coupled to mass spectrometry (LC–MS) and peptide mapping to understand post-translational modifications (PTMs) can be used to ensure that the correct sequence is present, the expected mass is observed and that any relevant PTMs are characterized. Of course, coupling this to the expected catalytic activity, in terms of converting the particular substrate(s) to the corresponding product(s), confirms that the protein that was expressed and purified is the correct one and is suitable for further study.
Purity is often a key consideration for biophysics (and structural) work, but is often, quite wrongly, under-appreciated in enzyme kinetics studies. Methods to assess purity include SDS or native PAGE and analytical gel filtration, as well as more biophysical approaches such as dynamic light scattering and analytical ultracentrifugation107. Usually, the criteria to confirm protein purity is the observation of a single band or peak, indicating that the protein is homogenous, monodisperse and in a single, defined oligomeric state. Coupling this information to enzyme kinetic data such as active site titration is critical, as the observed catalytic activity may arise from a small proportion of very active enzyme or a larger proportion of less active protein, and being able to distinguish between these differences is required to ensure that the protein is fully competent to bind ligands.
Concomitant to the measurements of catalytic rate, it is also important to measure the concentration of the enzyme, so that the activity can be carefully quantified and related to previous batches or similar enzymes. Typically, ultraviolet–visible spectrophotometry and/or Lowry, Bradford or bicinchoninic acid (BCA) assays108 are used to estimate protein concentrations, although one of the best, if somewhat laborious, methods is quantitative AAA109.
Although measuring total protein concentration is important, it must be understood that not all of the protein in the sample may be functional, in terms of binding to ligands or carrying out catalysis. Particularly useful in this respect may be biophysical binding methods, such as isothermal titration calorimetry and surface plasmon resonance (SPR)110. These methods allow reference ligands to be characterized in terms of affinity and other parameters, for example, enthalpy of binding, as well as in certain circumstances, the stoichiometry of the binding interaction. Assuming that the concentration of the ligand is accurately known, this can allow an estimation of the binding-competent component of the total protein concentration, which can be compared with functional measurements in catalytic assays. Comparison of parameters including K d, ΔH, stoichiometry, K m, k cat, k cat/K m and K i allows the evaluation of the functional protein in different preparations or across different proteins that may be important to assay during a drug discovery programme.
Other components in addition to the protein reagent can be evaluated in both biochemical and biophysical approaches before assay development. It is important to understand whether tool compounds highlighted in the literature behave as expected before using them as standard reference compounds in hit-finding campaigns. This is a crucial early activity, especially given that it is often difficult to reproduce published data111,112,113, and one that often takes relatively little effort but potentially results in high gain, reducing time spent on spurious tools or unwanted mechanisms in later phases.
Assay development. HTS assays are usually developed to search for chemical equity having the desired inhibitory effect via an appropriate mechanism. Combining biophysical measurements with enzyme kinetic data allows balanced or discriminatory assays to be developed, whereby a range of different mechanisms or a particular individual mechanism can be identified. This requires a thorough understanding of the mechanism of catalysis, which defines the order of addition of substrates and the release of products. Biophysical methods allow the characterization of binding affinities of substrates and products to a range of different enzyme forms (usually as long as a catalytically competent species is not formed), which can often facilitate more detailed multivariate enzyme kinetic studies. This information can, for instance, be used to adjust assay concentrations of substrates to bias towards a particular type of inhibition mechanism (for example, high concentrations of substrate bias away from weak substrate-competitive inhibitors).
Biophysical methods, such as SPR or NMR, are also employed at AstraZeneca as an orthogonal approach to evaluate hits found during pre-screening of a small number of compounds designed, as much as possible, to represent the diversity of the full screening deck114. The aim of this pre-screening activity is to give an initial assessment of the likely hit rate, assess factors including false positive and negative rates, identify potential technology interference, evaluate reproducibility and gauge the likelihood of other assay artefacts, such as plate pattern effects.
The number of hits expected in this process is usually low enough for biophysical methods to evaluate them all for binding to the target protein. The focus of this activity is to inform on the possible reasons for generating false positives, leading to improvements in the assay methodology or the screening cascade and sometimes the discontinuation of planned screens115.
Hit evaluation. The purpose of the hit-evaluation process is to identify those hits that elicit their activity via a valid mechanism and to remove those compounds for which their primary screening activity is undesirable. Combining an enzyme assay-based primary screen with a biophysical-based follow-up assay has some advantages. For example, substrate turnover may be monitored using mass spectrometry or NMR-based methods to mitigate artefacts encountered within optical-based biochemical assays. Another advantage of biophysical methods is that they allow the direct verification of binding to the target protein, allowing problems such as binding to additional components of the enzyme assay to be ruled out. For example, compounds may bind to other protein components, such as capturing antibodies, coupling enzymes, artificial protein substrates or even fluorescent peptides116,117. The use of biophysical techniques to probe direct target engagement under a range of assay conditions can help avoid wasting time following spurious mechanisms.
Biophysical methods also can, at this early stage, provide additional information on the mode of action of the initial hits. For example, information on the binding specificity (to related or relevant off-target proteins) as well as the binding stoichiometry can be acquired relatively straightforwardly, and additional quantitative data on binding kinetics and thermodynamics are generated to afford an understanding of potential binding mechanisms.
Orthogonal approaches to lead generation
The goals of lead generation are to choose a suitable starting point for the chemistry, identify tools that will allow investigation of the biological hypothesis and use these tools to uncover issues with the identified chemical series. Subsequently, efforts are made to resolve those problems in order to develop one or two chemical series that will progress further. Thus, an understanding of SARs and the potential for further optimization of potency and selectivity that will drive compound design are key desirables during lead generation. Mechanistic enzymology has had a role in delivering this information for many years, and measurements of IC50 are still prominent in this process today. However, reliance on IC50 is problematic, as the values may change with the assay conditions118, and understanding the impact of changes in assay conditions on the measured binding affinity of the test compound allows for better predictions of cellular potency and in vivo effects. This becomes increasingly important when novel targets, novel mechanisms or new binding sites are the focus of the drug discovery programme, and understanding the cause of the differences between the K d or K i values and IC50 is perhaps more important than for well-characterized or established targets.
An example of this is in the characterization of compounds binding to allosteric sites119. A combination of biophysical methods and enzyme kinetic and structural studies allowed characterization of inhibitors of spleen tyrosine kinase (SYK), which were hypothesized to function by reinforcing the natural regulatory contacts between the SH2 and kinase domains. Understanding this novel mode of inhibition provides a potentially new opportunity for the design of compounds with improved selectivity profiles. In this study, following purity and concentration checks as described above, fluorescence binding studies were combined with analytical ultracentrifugation (AUC) and small-angle X-ray scattering, as well as detailed enzyme kinetic experiments in which the mechanism of inhibition was assessed for compounds inhibiting SYK activation by immunoreceptor tyrosine-based activation motif (ITAM) binding, LynB phosphorylation or autophosphorylation. The compound X1 was shown to inhibit SYK activation by all three activation mechanisms: autophosphorylation, LynB phosphorylation and ITAM binding. The compound showed non-competitive inhibition against ATP and peptide substrates, thus preventing autophosphorylation by trapping SYK in its inactive basal state. The observation of competitive inhibition with respect to LynB activation was postulated to be due to a direct overlap of binding sites on SYK, although a more complicated kinetic model could not be ruled out. Another study on the regulation of tyrosine kinase activity identified an allosteric network of dynamically coupled residues in proto-oncogene tyrosine protein kinase SRC that connect regulatory sites to the ATP and protein substrate-binding sites120. It was the combination of data for substrate analogues and product binding at different peptide concentrations from fluorescence anisotropy and isothermal titration calorimetry (ITC) experiments, along with the observation of negative cooperativity for peptide binding in the presence of ATP from enzyme kinetics studies, coupled with molecular dynamics simulations based on the kinase domain from an X-ray structure of the SRC system in an active conformation, which allowed this signal-relay mechanism to be characterized.
Conclusion
Molecular enzymology has come a long way since its foundations over a century ago121,122. Access to purified target enzymes in large quantities, utilizing recombinant expression technology, has permitted detailed characterization of mechanistic details in the absence and presence of inhibitors. The combination of these mechanistic studies with the increasing access to high-resolution individual protein and complex structures, along with biophysical methods that can help probe particular enzyme forms, has had a broad impact on the understanding of disease processes and their modulation.
It is not surprising that modern drug discovery has also moved forward substantially over the same timescale123, as the incisive hypotheses, concepts and methodical rigour of the enzymologist have been applied to scientific problems faced within drug discovery. As with many time-honoured disciplines, sometimes the value of such stringent studies can be overlooked as the sequential processes and timelines that industrial drug discovery often demands begin to dominate. Of course, the development of new medicines is a complex process, and application of the principles of detailed mechanistic enzymology will not solve all of the issues, but increased focus on scientific rigour at the early stages will provide a solid foundation for increased success.
The concept of focusing on science was exemplified recently when AstraZeneca published a framework founded on project quality and depth of understanding as a key driver of success124. This '5 Rs' framework (the right target, the right tissue, the right safety, the right patient and the right commercial potential) can be modified to encompass the influence of mechanistic enzymology in the early stages of drug discovery: right target + right reagents + right assay + right mechanism = right compound.
A detailed understanding of target biochemistry before hit identification allows the selection of more physiologically relevant reagents and assay formats and thus supports the identification of desired mechanisms by appropriate screening (allowing a choice among balanced, focused and discriminatory assays). It also underpins conclusions derived from detailed follow-up characterization once suitable compounds have been identified or designed. Additionally, it enhances the quality of systems biology modelling, enabling more detailed pathway analysis and quantitative interrogation of required compound characteristics.
Detailed understanding of the molecular interactions of test compounds enables the selection of truly differentiated series. Early prioritization of these studies thus facilitates the identification of unwanted mechanisms, allowing specific deprioritization of compounds that would otherwise delay development due to issues such as lack of true target engagement or action via mechanisms that are not suitable for progression. This mechanism-based, informed decision-making process ultimately leads to better-quality compounds with a greater chance of clinical success, enhances the quality of regulatory submissions and produces higher-quality publications.
Publisher's note
Springer Nature remains neutral with regard to jurisdictional claims in published maps and institutional affiliations.
Change history
-
15 December 2017
In the affiliations of this article, the address provided for Discovery Sciences, IMED Biotech Unit, AstraZeneca was incorrect and should be Building 310, Cambridge Science Park, Milton Road, Cambridge CB4 0WG, UK. The article has been corrected in the print and online version.
References
- 1
Imming, P., Sinning, C. & Meyer, A. Drugs, their targets and the nature and number of drug targets. Nat. Rev. Drug Discov. 5, 821–834 (2006).
Article CAS Google Scholar
- 2
Hopkins, A. L. & Groom, C. R. The druggable genome. Nat. Rev. Drug Discov. 1, 727–730 (2002).
Article CAS PubMed PubMed Central Google Scholar
- 3
Skeggs, L. T., Kahn, J. R. & Shumway, N. P. The preparation and function of the hypertensin-converting enzyme. J. Exp. Med. 103, 295–299 (1956).
Article CAS PubMed PubMed Central Google Scholar
- 4
Ondetti, M. A., Rubin, B. & Cushman, D. W. Design of specific inhibitors of angiotensin-converting enzyme: new class of orally active antihypertensive agents. Science 196, 441–444 (1977).
Article CAS Google Scholar
- 5
Cushman, D. W., Cheung, H., Sabo, E. & Ondetti, M. Design of potent competitive inhibitors of angiotensin-converting enzyme. Carboxyalkanoyl and mercapto-alkanoyl amino acids. Biochemistry 16, 5484–5491 (1977).
Article CAS Google Scholar
- 6
Patchett, A. A. et al. A new class of angiotensin-converting enzyme inhibitors. Nature 288, 280–283 (1980).
Article CAS Google Scholar
- 7
Roth, B. D. The discovery and development of atorvastatin, a potent novel hypolipidemic agent. Progress Med. Chem. 40, 1–22 (2002).
Article CAS Google Scholar
- 8
Alberts, A. et al. Mevinolin: a highly potent competitive inhibitor of hydroxymethylglutaryl-coenzyme A reductase and a cholesterol-lowering agent. Proc. Natl Acad. Sci. USA 77, 3957–3961 (1980).
Article CAS Google Scholar
- 9
Nakamura, C. E. & Abeles, R. H. Mode of interaction of β-hydroxy-β-methylglutaryl coenzyme A reductase with strong binding inhibitors: compactin and related compounds. Biochemistry 24, 1364–1376 (1985).
Article CAS Google Scholar
- 10
Roberts, N. A. et al. Rational design of peptide-based HIV proteinase inhibitors. Science 248, 358–362 (1990).
Article CAS Google Scholar
- 11
Vacca, J. et al. L-735,524: an orally bioavailable human immunodeficiency virus type 1 protease inhibitor. Proc. Natl Acad. Sci. USA 91, 4096–4100 (1994).
Article CAS Google Scholar
- 12
Meek, T. D. Inhibitors of HIV-1 protease. J. Enzyme Inhib. 6, 65–98 (1992).
Article CAS Google Scholar
- 13
Robertson, J. G. Mechanistic basis of enzyme-targeted drugs. Biochemistry 44, 5561–5571 (2005).
Article CAS Google Scholar
- 14
Patel, M. P. et al. Kinetic and chemical mechanisms of the fabG-encoded Streptococcus pneumoniae β-ketoacyl-ACP reductase. Biochemistry 44, 16753–16765 (2005).
Article CAS Google Scholar
- 15
Dryer, G. et al. Hydroxyethylene isostere inhibitors of HIV-1 protease structure-activity analysis using enzyme kinetics, X-ray crystallography, and infected T-cell assays. Biochemistry 31, 6646–6659 (1992).
Article Google Scholar
- 16
Schramm, V. L. Transition states, analogues, and drug development. ACS Chem. Biol. 8, 71–81 (2013).
Article CAS PubMed PubMed Central Google Scholar
- 17
Schramm, V. L. Transition states and transition state analogue interactions with enzymes. Accounts Chem. Res. 48, 1032–1039 (2015).
Article CAS Google Scholar
- 18
Pham, T. V. et al. Mechanism-based inactivator of isocitrate lyases 1 and 2 from Mycobacterium tuberculosis. Proc. Natl Acad. Sci. USA 114, 7617–7622 (2017).
Article CAS Google Scholar
- 19
De Cesco, S., Kurian, J., Dufresne, C., Mittermaier, A. & Moitessier, N. Covalent inhibitors design and discovery. Eur. J. Med. Chem. 138, 96–114 (2017).
Article CAS Google Scholar
- 20
Radzicka, A. & Wolfenden, R. Transition state and multisubstrate analog inhibitors. Methods Enzymol. 249, 284–312 (1995).
Article CAS Google Scholar
- 21
Byers, L. D. & Wolfenden, R. Binding of the by-product analog benzylsuccinic acid by carboxypeptidase A. Biochemistry 12, 2070–2078 (1973).
Article CAS Google Scholar
- 22
Copeland, R. A., Pompliano, D. L. & Meek, T. D. Drug-target residence time and its implications for lead optimization. Nat. Rev. Drug Discov. 5, 730–739 (2006). This seminal paper introduces an alternative approach to drug optimization, focusing on drug–target residence time rather than the optimization of thermodynamic affinity alone.
Article CAS Google Scholar
- 23
Overington, J. P., Al-Lazikani, B. & Hopkins, A. L. How many drug targets are there? Nat. Rev. Drug Discov. 5, 993–996 (2006).
Article CAS PubMed PubMed Central Google Scholar
- 24
Smith, G. F. 1-Medicinal chemistry by the numbers: the physicochemistry, thermodynamics and kinetics of modern drug design. Progress Med. Chem. 48, 1–29 (2009).
Article CAS Google Scholar
- 25
Sachsenmaier, C. & Schachtele, C. Integrated technology platform protein kinases for drug development in oncology. Biotechniques 33, S101–S106 (2002).
Article Google Scholar
- 26
Lindsley, C. W. et al. Allosteric Akt (PKB) inhibitors: discovery and SAR of isozyme selective inhibitors. Bioorg. Med. Chem. Lett. 15, 761–764 (2005).
Article CAS Google Scholar
- 27
Barnett, S. F. et al. Identification and characterization of pleckstrin-homology-domain-dependent and isoenzyme-specific Akt inhibitors. Biochem. J. 385, 399–408 (2005).
Article CAS PubMed PubMed Central Google Scholar
- 28
Joseph, R. E., Min, L. & Andreotti, A. H. The linker between SH2 and kinase domains positively regulates catalysis of the Tec family kinases. Biochemistry 46, 5455–5462 (2007).
Article CAS Google Scholar
- 29
Hays, J. L. & Watowich, S. J. Oligomerization-induced modulation of TPR-MET tyrosine kinase activity. J. Biol. Chem. 278, 27456–27463 (2003).
Article CAS Google Scholar
- 30
Timofeevski, S. L. et al. Enzymatic characterization of c-Met receptor tyrosine kinase oncogenic mutants and kinetic studies with aminopyridine and triazolopyrazine inhibitors. Biochemistry 48, 5339–5349 (2009).
Article CAS Google Scholar
- 31
Favata, M. F. et al. Identification of a novel inhibitor of mitogen-activated protein kinase kinase. J. Biol. Chem. 273, 18623–18632 (1998).
Article CAS PubMed PubMed Central Google Scholar
- 32
Solowiej, J. et al. Characterizing the effects of the juxtamembrane domain on vascular endothelial growth factor receptor-2 enzymatic activity, autophosphorylation, and inhibition by axitinib. Biochemistry 48, 7019–7031 (2009).
Article CAS Google Scholar
- 33
Kopcho, L. M. et al. Comparative studies of active site-ligand interactions among various recombinant constructs of human β-amyloid precursor protein cleaving enzyme. Arch. Biochem. Biophys. 410, 307–316 (2003).
Article CAS PubMed PubMed Central Google Scholar
- 34
Stevenson, L. M., Deal, M. S., Hagopian, J. C. & Lew, J. Activation mechanism of CDK2: role of cyclin binding versus phosphorylation. Biochemistry 41, 8528–8534 (2002).
Article CAS PubMed PubMed Central Google Scholar
- 35
Anderson, K. et al. Binding of TPX2 to Aurora A alters substrate and inhibitor interactions. Biochemistry 46, 10287–10295 (2007).
Article CAS PubMed PubMed Central Google Scholar
- 36
Kuzmichev, A., Nishioka, K., Erdjument-Bromage, H., Tempst, P. & Reinberg, D. Histone methyltransferase activity associated with a human multiprotein complex containing the Enhancer of Zeste protein. Genes Dev. 16, 2893–2905 (2002).
Article CAS PubMed PubMed Central Google Scholar
- 37
Dang, L. et al. Cancer-associated IDH1 mutations produce 2-hydroxyglutarate. Nature 462, 739–744 (2009).
Article CAS PubMed PubMed Central Google Scholar
- 38
Smits, A. H., Jansen, P. W., Poser, I., Hyman, A. A. & Vermeulen, M. Stoichiometry of chromatin-associated protein complexes revealed by label-free quantitative mass spectrometry-based proteomics. Nucleic Acids Res. 41, e28 (2013).
Article CAS Google Scholar
- 39
Krishnaswamy, S. & Betz, A. Exosites determine macromolecular substrate recognition by prothrombinase. Biochemistry 36, 12080–12086 (1997).
Article CAS PubMed PubMed Central Google Scholar
- 40
Harpel, M. R. et al. Mutagenesis and mechanism-based inhibition of Streptococcus pyogenes Glu-tRNAGln amidotransferase implicate a serine-based glutaminase site. Biochemistry 41, 6398–6407 (2002).
Article CAS Google Scholar
- 41
Szafranska, A. E. & Dalby, K. N. Kinetic mechanism for p38 MAP kinase α. A partial rapid-equilibrium random-order ternary-complex mechanism for the phosphorylation of a protein substrate. FEBS J. 272, 4631–4645 (2005).
Article CAS Google Scholar
- 42
LoGrasso, P. V. et al. Kinetic mechanism for p38 MAP kinase. Biochemistry 36, 10422–10427 (1997).
Article CAS Google Scholar
- 43
Chen, G., Porter, M. D., Bristol, J. R., Fitzgibbon, M. J. & Pazhanisamy, S. Kinetic mechanism of the p38-α MAP kinase: phosphoryl transfer to synthetic peptides. Biochemistry 39, 2079–2087 (2000).
Article CAS Google Scholar
- 44
Gao, X. & Harris, T. K. Steady-state kinetic mechanism of PDK1. J. Biol. Chem. 281, 21670–21681 (2006).
Article CAS PubMed PubMed Central Google Scholar
- 45
Keshwani, M. M., Gao, X. & Harris, T. K. Mechanism of PDK1-catalyzed Thr-229 phosphorylation of the S6K1 protein kinase. J. Biol. Chem. 284, 22611–22624 (2009).
Article CAS PubMed PubMed Central Google Scholar
- 46
Davidson, W. et al. Discovery and characterization of a substrate selective p38α inhibitor. Biochemistry 43, 11658–11671 (2004).
Article CAS Google Scholar
- 47
Pargellis, C. et al. Inhibition of p38 MAP kinase by utilizing a novel allosteric binding site. Nat. Struct. Mol. Biol. 9, 268 (2002).
Article CAS Google Scholar
- 48
Li, Y. et al. The target of the NSD family of histone lysine methyltransferases depends on the nature of the substrate. J. Biol. Chem. 284, 34283–34295 (2009).
Article CAS PubMed PubMed Central Google Scholar
- 49
Poulin, M. B. et al. Transition state for the NSD2-catalyzed methylation of histone H3 lysine 36. Proc. Natl Acad. Sci. 113, 1197–1201 (2016).
Article CAS Google Scholar
- 50
Bar-Even, A. et al. The moderately efficient enzyme: evolutionary and physicochemical trends shaping enzyme parameters. Biochemistry 50, 4402–4410 (2011).
Article CAS Google Scholar
- 51
Garuti, L., Roberti, M. & Bottegoni, G. Non-ATP competitive protein kinase inhibitors. Curr. Med. Chem. 17, 2804–2821 (2010).
Article CAS Google Scholar
- 52
Cornish-Bowden, A. Why is uncompetitive inhibition so rare?: A possible explanation, with implications for the design of drugs and pesticides. FEBS Lett. 203, 3–6 (1986). This paper provides insight into why uncompetitive inhibition is rarely encountered and suggests that it is a useful approach, as these inhibitors may have greater pharmacological effects.
Article CAS Google Scholar
- 53
Hedstrom, L. IMP dehydrogenase: structure, mechanism, and inhibition. Chem. Rev. 109, 2903–2928 (2009).
Article CAS PubMed PubMed Central Google Scholar
- 54
Ward, W. H. et al. Kinetic and structural characteristics of the inhibition of enoyl (acyl carrier protein) reductase by triclosan. Biochemistry 38, 12514–12525 (1999).
Article CAS Google Scholar
- 55
Swinney, D. C. Biochemical mechanisms of drug action: what does it take for success? Nat. Rev. Drug Discov. 3, 801–808 (2004).
Article CAS Google Scholar
- 56
Dahl, G. & Akerud, T. Pharmacokinetics and the drug-target residence time concept. Drug Discov. Today 18, 697–707 (2013). This paper demonstrates that the extension of binding due to a long drug–target residence time occurs only when the binding dissociation rate is slower than the PK elimination rate. Exemplary data for many drugs and/or drug candidates indicate that the opposite scenario is commonly observed.
Article CAS Google Scholar
- 57
Walkup, G. K. et al. Translating slow-binding inhibition kinetics into cellular and in vivo effects. Nat. Chem. Biol. 11, 416–423 (2015). This paper describes a mechanistic pharmacodynamic model that includes drug–target kinetic parameters. It has been applied to predict dose–response curves for inhibitors in an animal model of infection.
Article CAS PubMed PubMed Central Google Scholar
- 58
Johnson, D. S., Weerapana, E. & Cravatt, B. F. Strategies for discovering and derisking covalent, irreversible enzyme inhibitors. Future Med. Chem. 2, 949–964 (2010).
Article CAS PubMed PubMed Central Google Scholar
- 59
Copeland, R. A. Mechanistic considerations in high-throughput screening. Anal. Biochem. 320, 1–12 (2003). This paper describes the steps required to design the best hit-finding assay possible, taking into account the requirements for a successful screening campaign and also post-screening lead evaluation.
Article CAS Google Scholar
- 60
Segal, I. H. Enzyme Kinetics: Behavior and Analysis of Rapid Equilibrium and Steady-State Enzyme Systems (Wiley, 1975). This book is an essential text for any academic or industrial enzymologist, as it provides a comprehensive introduction to enzyme kinetics.
Google Scholar
- 61
Schneck, J. L. et al. Chemical mechanism of a cysteine protease, cathepsin C, as revealed by integration of both steady-state and pre-steady-state solvent kinetic isotope effects. Biochemistry 47, 8697–8710 (2008).
Article CAS Google Scholar
- 62
Noble, M. et al. The kinetic model of the shikimate pathway as a tool to optimize enzyme assays for high-throughput screening. Biotechnol. Bioeng. 95, 560–573 (2006).
Article CAS Google Scholar
- 63
Schneck, J. L. et al. Kinetic mechanism and rate-limiting steps of focal adhesion kinase-1. Biochemistry 49, 7151–7163 (2010).
Article CAS Google Scholar
- 64
Teague, S. J. Implications of protein flexibility for drug discovery. Nat. Rev. Drug Discov. 2, 527–541 (2003).
Article CAS Google Scholar
- 65
Thorne, N., Auld, D. S. & Inglese, J. Apparent activity in high-throughput screening: origins of compound-dependent assay interference. Curr. Opin. Chem. Biol. 14, 315–324 (2010).
Article CAS PubMed PubMed Central Google Scholar
- 66
Hermann, J. C. et al. Metal impurities cause false positives in high-throughput screening campaigns. ACS Med. Chem. Lett. 4, 197–200 (2013).
Article CAS Google Scholar
- 67
Di, L. & Kerns, E. H. Biological assay challenges from compound solubility: strategies for bioassay optimization. Drug Discov. Today 11, 446–451 (2006).
Article CAS Google Scholar
- 68
McGovern, S. L., Helfand, B. T., Feng, B. & Shoichet, B. K. A specific mechanism of nonspecific inhibition. J. Med. Chem. 46, 4265–4272 (2003). This paper shows that aggregates formed by promiscuous compounds can reversibly sequester enzymes, resulting in apparent inhibition. It presents post-screening approaches for detecting and avoiding such compounds.
Article CAS Google Scholar
- 69
Johnston, P. A. Redox cycling compounds generate H2O2 in HTS buffers containing strong reducing reagents — real hits or promiscuous artifacts? Curr. Opin. Chem. Biol. 15, 174–182 (2011).
Article CAS Google Scholar
- 70
Dahlin, J. L., Baell, J. & Walters, M. A. in Assay Guidance Manual (eds Sittampalam, G. S. et al.) (2004).
Google Scholar
- 71
McGovern, S. L. & Shoichet, B. K. Kinase inhibitors: not just for kinases anymore. J. Med. Chem. 46, 1478–1483 (2003).
Article CAS Google Scholar
- 72
Baell, J. B. & Holloway, G. A. New substructure filters for removal of pan assay interference compounds (PAINS) from screening libraries and for their exclusion in bioassays. J. Med. Chem. 53, 2719–2740 (2010).
Article CAS PubMed PubMed Central Google Scholar
- 73
Baell, J. & Walters, M. A. Chemical con artists foil drug discovery. Nature 513, 481 (2014).
Article CAS Google Scholar
- 74
Nissink, J. W. M. & Blackburn, S. Quantification of frequent-hitter behavior based on historical high-throughput screening data. Future Med. Chem. 6, 1113–1126 (2014).
Article CAS Google Scholar
- 75
Saubern, S., Guha, R. & Baell, J. B. KNIME workflow to assess PAINS filters in SMARTS format. Comparison of RDKit and Indigo cheminformatics libraries. Mol. Informat. 30, 847–850 (2011).
Article CAS Google Scholar
- 76
Shoichet, B. K. Interpreting steep dose-response curves in early inhibitor discovery. J. Med. Chem. 49, 7274–7277 (2006).
Article CAS Google Scholar
- 77
Shoichet, B. K. Screening in a spirit haunted world. Drug Discov. Today 11, 607–615 (2006).
Article CAS PubMed PubMed Central Google Scholar
- 78
Selwyn, M. J. A simple test for inactivation of an enzyme during assay. Biochim. Biophys. Acta 105, 193–195 (1965).
Article CAS Google Scholar
- 79
Copeland, R. A. in Enzymes: A Practical Introduction to Structure, Mechanism, and Data Analysis 2nd edn (ed. Copeland, R. A. ) 266–304 (Wiley, 2002).
Google Scholar
- 80
Singh, J., Petter, R. C., Baillie, T. A. & Whitty, A. The resurgence of covalent drugs. Nat. Rev. Drug Discov. 10, 307–317 (2011).
Article CAS PubMed PubMed Central Google Scholar
- 81
Rawat, R., Whitty, A. & Tonge, P. J. The isoniazid-NAD adduct is a slow, tight-binding inhibitor of InhA, the Mycobacterium tuberculosis enoyl reductase: adduct affinity and drug resistance. Proc. Natl Acad. Sci. USA 100, 13881–13886 (2003).
Article CAS Google Scholar
- 82
Garvey, E. P. et al. Potent inhibitors of HIV-1 integrase display a two-step, slow-binding inhibition mechanism which is absent in a drug-resistant T66I/M154I mutant. Biochemistry 48, 1644–1653 (2009).
Article CAS Google Scholar
- 83
Rudolph, J., Xiao, Y., Pardi, A. & Ahn, N. G. Slow inhibition and conformation selective properties of extracellular signal-regulated kinase 1 and 2 inhibitors. Biochemistry 54, 22–31 (2014).
Article CAS PubMed PubMed Central Google Scholar
- 84
Sculley, M. J., Morrison, J. F. & Cleland, W. W. Slow-binding inhibition: the general case. Biochim. Biophys. Acta 1298, 78–86 (1996).
Article Google Scholar
- 85
Morrison, J. F. & Walsh, C. T. The behavior and significance of slow-binding enzyme inhibitors. Adv. Enzymol. Relat. Areas Mol. Biol. 61, 201–301 (1988). This article describes the detection and analysis of slow-binding inhibition.
CAS PubMed Google Scholar
- 86
Copeland, R. A., Basavapathruni, A., Moyer, M. & Scott, M. P. Impact of enzyme concentration and residence time on apparent activity recovery in jump dilution analysis. Anal. Biochem. 416, 206–210 (2011).
Article CAS Google Scholar
- 87
Copeland, R. A., Lombardo, D., Giannaras, J. & Decicco, C. P. Estimating KI values for tight binding inhibitors from dose-response plots. Bioorg. Med. Chem. Lett. 5, 1947–1952 (1995).
Article CAS Google Scholar
- 88
Goldstein, A. The mechanism of enzyme-inhibitor-substrate reactions: illustrated by the cholinesterase-physostigmine-acetylcholine system. J. Gen. Physiol. 27, 529–580 (1944).
Article CAS PubMed PubMed Central Google Scholar
- 89
Morrison, J. F. Kinetics of the reversible inhibition of enzyme-catalysed reactions by tight-binding inhibitors. Biochim. Biophys. Acta 185, 269–286 (1969). This paper highlights the challenges associated with the determination of inhibition constants for tight-binding inhibitors and presents the theory behind the analysis.
Article CAS Google Scholar
- 90
Murphy, D. J. Determination of accurate KI values for tight-binding enzyme inhibitors: an in silico study of experimental error and assay design. Anal. Biochem. 327, 61–67 (2004).
Article CAS Google Scholar
- 91
Copeland, R. A., Harpel, M. R. & Tummino, P. J. Targeting enzyme inhibitors in drug discovery. Expert Opin. Ther. Targets 11, 967–978 (2007).
Article CAS Google Scholar
- 92
Bauer, R. A. Covalent inhibitors in drug discovery: from accidental discoveries to avoided liabilities and designed therapies. Drug Discov. Today 20, 1061–1073 (2015).
Article CAS Google Scholar
- 93
Strelow, J. M. A. Perspective on the kinetics of covalent and irreversible inhibition. SLAS Discov. 22, 3–20 (2017). This paper provides a detailed explanation of the characterization of irreversible inhibition.
CAS PubMed Google Scholar
- 94
Krippendorff, B. F., Neuhaus, R., Lienau, P., Reichel, A. & Huisinga, W. Mechanism-based inhibition: deriving KI and kinact directly from time-dependent IC50 values. J. Biomol. Screen 14, 913–923 (2009).
Article CAS Google Scholar
- 95
Jo¨st, C., Nitsche, C., Scholz, T., Roux, L. & Klein, C. D. Promiscuity and selectivity in covalent enzyme inhibition: a systematic study of electrophilic fragments. J. Med. Chem. 57, 7590–7599 (2014).
Article CAS Google Scholar
- 96
Lanning, B. R. et al. A road map to evaluate the proteome-wide selectivity of covalent kinase inhibitors. Nat. Chem. Biol. 10, 760–767 (2014).
Article CAS PubMed PubMed Central Google Scholar
- 97
Schwartz, P. A. et al. Covalent EGFR inhibitor analysis reveals importance of reversible interactions to potency and mechanisms of drug resistance. Proc. Natl Acad. Sci. USA 111, 173–178 (2014).
Article CAS Google Scholar
- 98
Ring, B., Wrighton, S. A. & Mohutsky, M. in Enzyme Kinetics in Drug Metabolism: Fundamentals and Applications (eds Nagar, S., Argikar, U. & Tweedie, D.) 37–56 (Springer, 2014).
Book Google Scholar
- 99
Fleming, M. A. et al. Inhibition of IMPDH by mycophenolic acid: dissection of forward and reverse pathways using capillary electrophoresis. Biochemistry 35, 6990–6997 (1996).
Article CAS Google Scholar
- 100
Hedstrom, L. & Wang, C. C. Mycophenolic acid and thiazole adenine dinucleotide inhibition of Tritrichomonas foetus inosine 5′-monophosphate dehydrogenase: implications on enzyme mechanism. Biochemistry 29, 849–854 (1990).
Article CAS Google Scholar
- 101
Copeland, R. A. Evaluation of Enzyme Inhibitors in Drug Discovery: A Guide for Medicinal Chemists and Pharmacologists (John Wiley & Sons, 2013).
Book Google Scholar
- 102
Munson, P. J. & Rodbard, D. An exact correction to the "Cheng-Prusoff" correction. J. Recept. Res. 8, 533–546 (1988). This paper describes the effect of the concentration of labelled drug and the concentration of the binding site on the measured ED 50 and hence K i values, as well as provides an explicit correction to overcome these issues.
Article CAS Google Scholar
- 103
Cheng, H. C. The influence of cooperativity on the determination of dissociation constants: examination of the Cheng-Prusoff equation, the Scatchard analysis, the Schild analysis and related power equations. Pharmacol. Res. 50, 21–40 (2004).
Article CAS Google Scholar
- 104
Johnson, K. A. A century of enzyme kinetic analysis, 1913 to 2013. FEBS Lett. 587, 2753–2766 (2013).
Article CAS PubMed PubMed Central Google Scholar
- 105
Cornish-Bowden, A. Analysis and interpretation of enzyme kinetic data. Persp. Sci. 1, 121–125 (2014).
Google Scholar
- 106
Renaud, J. P. et al. Biophysics in drug discovery: impact, challenges and opportunities. Nat. Rev. Drug Discov. 15, 679–698 (2016).
Article CAS Google Scholar
- 107
Holdgate, G. et al. in Protein-Ligand Interactions: Methods and Applications (eds Williams, M. & Daviter, T.) 327–355 (Springer, 2013).
Book Google Scholar
- 108
Olson, B. J. & Markwell, J. UNIT 3.4: Assays for determination of protein concentration. Curr. Protoc. Protein Sci. http://dx.doi.org/10.1002/0471140864.ps0304s48 (2007).
- 109
Dunn, B. UNIT 3.2: Quantitative amino acid analysis. Curr. Protoc. Protein Sci. http://dx.doi.org/10.1002/0471140864.ps0302s00 (2001).
- 110
Holdgate, G. A., Anderson, M., Edfeldt, F. & Geschwindner, S. Affinity-based, biophysical methods to detect and analyze ligand binding to recombinant proteins: matching high information content with high throughput. J. Struct. Biol. 172, 142–157 (2010).
Article CAS Google Scholar
- 111
Prinz, F., Schlange, T. & Asadullah, K. Believe it or not: how much can we rely on published data on potential drug targets? Nat. Rev. Drug Discov. 10, 712 (2011).
Article CAS Google Scholar
- 112
Frye, S. V. et al. Tackling reproducibility in academic preclinical drug discovery. Nat. Rev. Drug Discov. 14, 733–734 (2015).
Article CAS Google Scholar
- 113
Mullane, K. & Williams, M. Unknown unknowns in biomedical research: does an inability to deal with ambiguity contribute to issues of irreproducibility? Biochem. Pharmacol. 97, 133–136 (2015).
Article CAS Google Scholar
- 114
Edfeldt, F. N., Folmer, R. H. & Breeze, A. L. Fragment screening to predict druggability (ligandability) and lead discovery success. Drug Discov. Today 16, 284–287 (2011).
Article CAS Google Scholar
- 115
Folmer, R. H. Integrating biophysics with HTS-driven drug discovery projects. Drug Discov. Today 21, 491–498 (2016).
Article CAS Google Scholar
- 116
Kaeberlein, M. et al. Substrate-specific activation of sirtuins by resveratrol. J. Biol. Chem. 280, 17038–17045 (2005).
Article CAS Google Scholar
- 117
Borra, M. T., Smith, B. C. & Denu, J. M. Mechanism of human SIRT1 activation by resveratrol. J. Biol. Chem. 280, 17187–17195 (2005).
Article CAS Google Scholar
- 118
Kalliokoski, T., Kramer, C., Vulpetti, A. & Gedeck, P. Comparability of mixed IC50 data – a statistical analysis. PloS ONE 8, e61007 (2013).
Article CAS PubMed PubMed Central Google Scholar
- 119
Hall, J. et al. Biophysical and mechanistic insights into novel allosteric inhibitor of spleen tyrosine kinase. J. Biol. Chem. 287, 7717–7727 (2012).
Article CAS PubMed PubMed Central Google Scholar
- 120
Foda, Z. H., Shan, Y., Kim, E. T., Shaw, D. E. & Seeliger, M. A. A dynamically coupled allosteric network underlies binding cooperativity in Src kinase. Nat. Commun. 6, 5939 (2015).
Article CAS PubMed PubMed Central Google Scholar
- 121
Brown, A. J. XXXVI.—Enzyme action. J. Chem. Soc., Trans. 81, 373–388 (1902).
Article CAS Google Scholar
- 122
Michaelis, L. & Menten, M. L. Die kinetik der invertinwirkung. Biochem. Z. 49, 333–369 (1913).
CAS Google Scholar
- 123
Ehrlich, P. Address in pathology on chemiotherapy: delivered before the Seventeenth International Congress of Medicine. Br. Med. J. 2, 353–359 (1913).
Article CAS PubMed PubMed Central Google Scholar
- 124
Cook, D. et al. Lessons learned from the fate of AstraZeneca's drug pipeline: a five-dimensional framework. Nat. Rev. Drug Discov. 13, 419–431 (2014).
Article CAS Google Scholar
- 125
Mezzasalma, T. M. et al. Enhancing recombinant protein quality and yield by protein stability profiling. J. Biomol. Screen 12, 418–428 (2007).
Article CAS Google Scholar
- 126
Hajduk, P. J. et al. A strategy for high-throughput assay development using leads derived from nuclear magnetic resonance-based screening. J. Biomol. Screen 7, 429–432 (2002).
Article CAS Google Scholar
- 127
Hajduk, P. J. & Burns, D. J. Integration of NMR and high-throughput screening. Comb. Chem. High Throughput Screen 5, 613–621 (2002).
Article CAS Google Scholar
- 128
Genick, C. C. et al. Applications of biophysics in high-throughput screening hit validation. J. Biomol. Screen 19, 707–714 (2014).
Article CAS Google Scholar
- 129
Evenas, J. et al. HTS followed by NMR based counterscreening. Discovery and optimization of pyrimidones as reversible and competitive inhibitors of xanthine oxidase. Bioorg. Med. Chem. Lett. 24, 1315–1321 (2014).
Article CAS Google Scholar
- 130
Ciulli, A. Biophysical screening for the discovery of small-molecule ligands. Methods Mol. Biol. 1008, 357–388 (2013).
Article CAS PubMed PubMed Central Google Scholar
- 131
Geschwindner, S. in Lead Generation: Methods and Strategies Ch. 14 (ed. Holenz, J. ) (Wiley, 2016).
Google Scholar
- 132
Bergsdorf, C. & Ottl, J. Affinity-based screening techniques: their impact and benefit to increase the number of high quality leads. Expert Opin. Drug Discov. 5, 1095–1107 (2010).
Article Google Scholar
- 133
Huber, W. A new strategy for improved secondary screening and lead optimization using high-resolution SPR characterization of compound-target interactions. J. Mol. Recognit. 18, 273–281 (2005).
Article CAS Google Scholar
- 134
Barsyte-Lovejoy, D. et al. (R)-PFI-2 is a potent and selective inhibitor of SETD7 methyltransferase activity in cells. Proc. Natl Acad. Sci. USA 111, 12853–12858 (2014).
Article CAS Google Scholar
- 135
Davis, M. I. et al. Biochemical, cellular, and biophysical characterization of a potent inhibitor of mutant isocitrate dehydrogenase IDH1. J. Biol. Chem. 289, 13717–13725 (2014).
Article CAS PubMed PubMed Central Google Scholar
Download references
Acknowledgements
The authors would like to thank S. Thrall for critical input into Fig. 1.
Ethics declarations
Competing interests
G.H. and R.G. are current employees of AstraZeneca. T.M. is a former employee of GSK.
PowerPoint slides
Glossary
- Mechanism
-
A process by which a reaction takes place, fully determined when all the intermediates, complexes and conformational states of an enzyme are characterized and the rate constants associated with the conversion between them are quantified. The term is often used in inhibition studies to distinguish between different modes of inhibition.
- Half-maximal inhibitory concentration
-
(IC50). The inhibitor concentration that gives a 50% decrease in rate under the specific assay conditions employed.
- Random-order mechanism
-
A reaction mechanism in which either of the two substrates may bind to the enzyme first to form a binary complex, followed by the other to form a ternary complex.
- Non-competitive inhibitors
-
Inhibitors that bind with equal affinity both before and after the varied substrate.
- Uncompetitive inhibitors
-
Inhibitors that bind only after the substrate.
- Slow-binding inhibition
-
An inhibition that occurs slowly on the timescale of the assay as the enzyme–inhibitor complex concentration increases to its steady-state level.
- Competitive inhibition
-
A type of inhibition where the inhibitor binds only before the varied substrate (see also non-competitive and uncompetitive inhibition).
- Reversible inhibition
-
An inhibition that can be reversed on the timescale of the assay by competition or dilution. Reversible inhibitors are not precluded from forming covalent bonds with the enzyme.
- Irreversible inhibition
-
An inhibition that cannot be reversed on the timescale of the assay. Truly irreversible inhibitors never dissociate from the enzyme and are characterized by an inactivation rate constant, not a dissociation constant. Irreversible inhibitors do not necessarily have to be covalently bound.
- Tight-binding inhibition
-
A type of inhibition occurring under conditions when the concentration of inhibitor required to cause inhibition is similar to the enzyme concentration, leading to depletion of the free inhibitor concentration. This results in breakdown of the usual assumptions leading to simple inhibition kinetics and requires a more complex rate equation.
- Mixed inhibition
-
A type of inhibition in which the inhibitor binds both before and after the varied substrate.
Rights and permissions
About this article
Cite this article
Holdgate, G., Meek, T. & Grimley, R. Mechanistic enzymology in drug discovery: a fresh perspective. Nat Rev Drug Discov 17, 115–132 (2018). https://doi.org/10.1038/nrd.2017.219
Download citation
-
Published:
-
Issue Date:
-
DOI : https://doi.org/10.1038/nrd.2017.219
Further reading
How Are Enzyme Activity Assays Used for Drug Design
Source: https://www.nature.com/articles/nrd.2017.219
0 Response to "How Are Enzyme Activity Assays Used for Drug Design"
Enregistrer un commentaire